Identifying High-Risk Patients Early
One of the biggest challenges facing integrated health systems and risk-bearing entities (whether payers or providers) is figuring out who is at high risk for hospitalization before the crisis happens. The trick is to have a tool that is sensitive enough to identify most of the target population, but specific enough to focus on those who can benefit from supportive interventions. It is increasingly clear that any program’s (this means us, palliative care teams!) ability to improve care value is critically reliant on targeting the right patients—not too many, not too few, but the right ones. In general, the categories of data used to identify the target population have relied upon claims data both for diagnoses and past utilization as predictors of future utilization. Putting aside the fact that most providers cannot generally access these data, past utilization and a bad diagnosis do not, by themselves, reliably predict future utilization. The inadequacy of this approach becomes obvious when we look at Melissa Aldridge and Amy Kelley’s analyses of the costliest 5% of patients in the U.S., fully half of whom have one time high costs, and then return to normal spending when they recover.
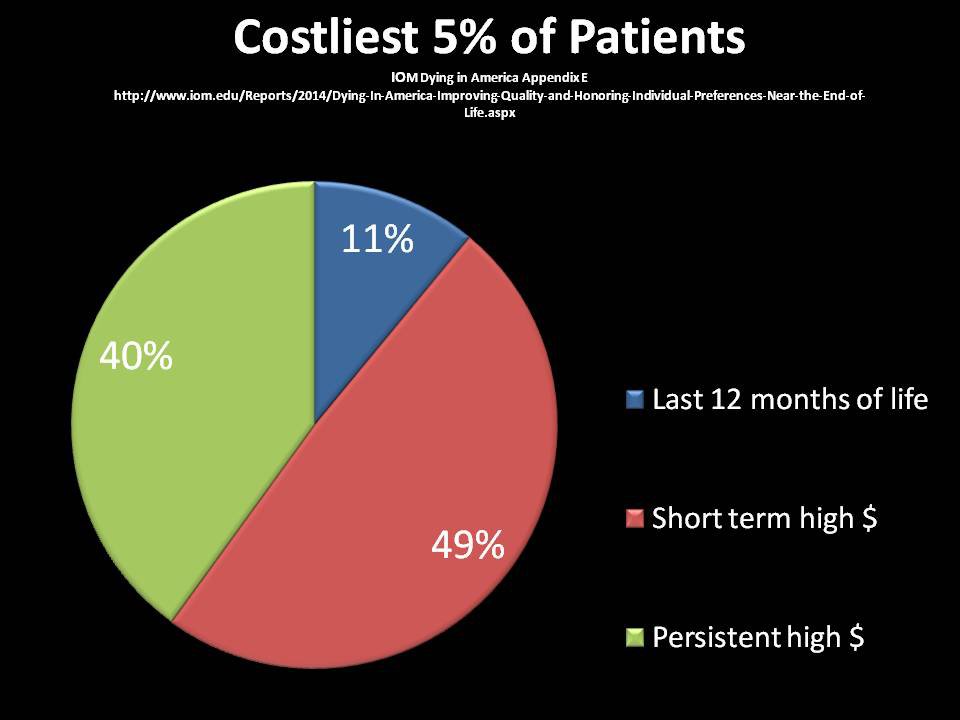
Consider, for example, a patient on hemodialysis three days a week who receives a successful transplant, or a patient with severe community-acquired pneumonia requiring an ICU and rehab stay who then goes on to a full functional recovery. Neither of these patients needs specialized palliative care or other services specifically designed for complex high-risk and high-cost populations, but their diagnostic codes and utilization data would place them—wrongly—in the target group.
Insurers routinely use predictive analytics, most of which are proprietary and not publicly available, to find high risk members who need care management. Since 2004, CMS has used something called hierarchical condition categories (HCCs) which rely on diagnostic codes in claims data paired with a complex statistical algorithm that enables adjustment of capitation rates for beneficiaries in Medicare Advantage (MA) plans. Complete and accurate documentation and coding helps MA plans maximize their reimbursement, and there is a growing cottage industry devoted to helping MA plans and their in-network providers to do just that. However, the Affordable Care Act, and, more recently, the Medicare Access and CHIP Reauthorization Act (MACRA), have accelerated the rise in assumption of financial risk by ALL front-line clinicians and health organizations. This means that all of us—not just insurers—need reliable, feasible and inexpensive algorithms to first identify and then support those patients at greatest risk. (Read more about MACRA and Medicare Payment Reform.) Clinicians practicing in all care settings, (e.g., hospitals and health systems, home care agencies, dialysis units, cancer centers and nursing homes) are all, or soon will be, held accountable for value (remember quality over cost!) through reimbursement rewards and penalties. In order to survive this new payment landscape, we must a. know our population and b. match services to their needs.
So it was with relief and excitement that I read Amy Kelley and colleagues’ recent paper in Health Services Research (HSR) titled “Identifying Older Adults with Serious Illness: A Critical Step toward Improving the Value of Health Care”. Using the Health and Retirement Study (HRS) (a nationally representative longitudinal cohort of adults over 50), the authors built on a consensus definition of serious illness (Kelley 2014) as follows:
“Serious illness is a condition that carries a high risk of mortality, negatively impacts quality of life and daily function, and/or is burdensome in symptoms, treatments, or caregiver stress.”
They then created three sub-definitions of serious illness, ranging from more sensitive (inclusive) for screening purposes to more specific (highest risk group) for intervention purposes. This required operationalizing the following terms: “serious medical conditions”, defined as those with a high risk of mortality, including such diagnoses as advanced cancer, heart failure, ESRD and dementia; “functional impairment”, defined as dependency in one or more activities of daily living; and “utilization”, defined as one or more hospitalizations in the last year or residing in a nursing home. The benefit of using these factors is that all are relatively accessible in the clinical setting (especially if we start requiring functional assessment in the EHR). The three sub-definitions were as follows (from broad to most restrictive):
- Serious condition and/or functional impairment (moderate risk, may benefit from screening for needs amenable to specialized services);
- Serious condition and/or functional impairment AND utilization (moderate-high risk, may benefit from needs assessment/specialized services);
- Serious condition AND functional impairment AND utilization (highest risk group, may benefit from specialist services)
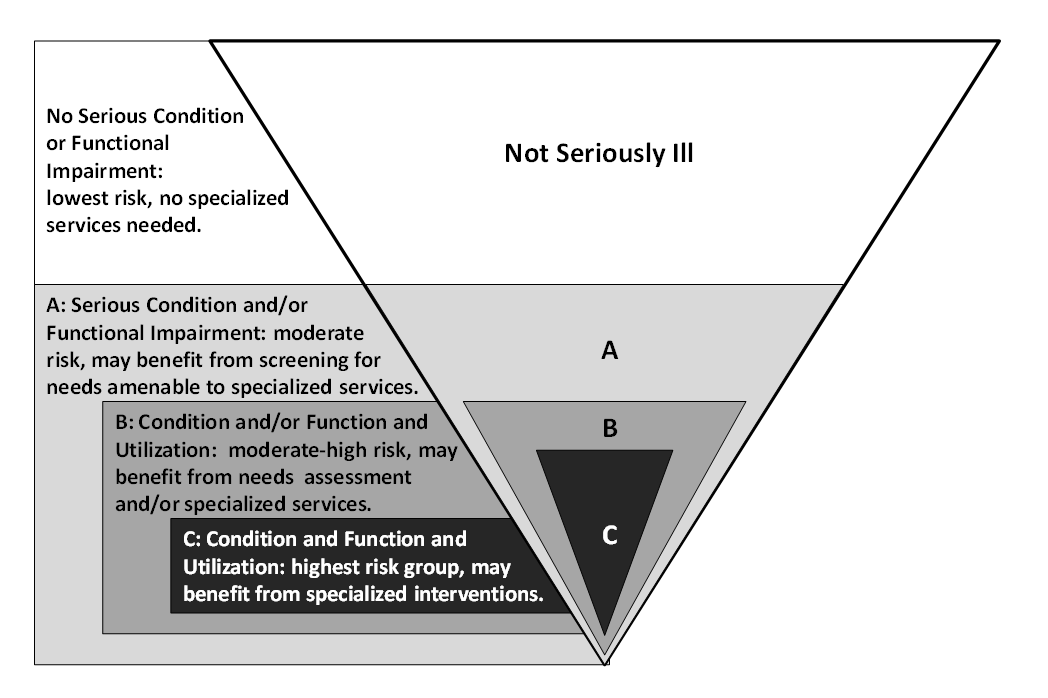
Source: Health Services Research
Among the 11,577 eligible HRS subjects, 5,297 fell into category A., 3,151 fell into category B., and 1,447 fell into category C. In comparison to the rest of the cohort, subjects in these three groups were more likely to be older, female, racially and ethnically diverse, less likely to be married, less wealthy, less educated and more likely to self-report fair or poor health. One year outcomes for each group were as follows:
1-year outcomes |
Not Seriously Ill (or “Comparison group”) |
Category A Serious condition and/or functional impairment |
Category B Serious condition and/or functional impairment AND utilization |
Category C Serious condition AND functional impairment AND utilization |
---|---|---|---|---|
Number of Subjects | 6,280 | 5,297 | 3,151 | 1,447 |
Hospitalization | 12% | 33% | 44% | 47% |
Total Medicare costs (mean) | $7,789 | $20,566 | $26,349 | $30,828 |
Mortality | 2% | 13% | 19% | 28% |
Wow. People in Category C (one or more serious illnesses and functional dependency and at least one hospital or nursing home stay in the preceding year) have a 47% chance of being hospitalized in the coming year, have 4 times the Medicare spending of those who did not fall under any category and experience a 28% mortality rate. That means that 72% of the patients in Group C did not die in the subsequent year, further underscoring the importance of operational screening criteria that do not rely on an accurate prognosis.
So how can those of us on the front lines operationalize these criteria? Talk to your quality colleagues about adding a mandatory functional status field to your EHR in all care settings. Identify those in your population with at least one serious medical condition. And get a list of everyone who has been hospitalized or in a nursing home in the prior year among both of these groups. Implementing these changes will help ensure that your programs identify the patients most likely to benefit from palliative care, without casting the net so wide that it exceeds your capacity. Of course that leaves the question of what to do once you have identified and then risk-stratified these groups . . . but that’s for another blog post!